With LaunchDarkly, we make it easy to add feature flags at scale to any application across any platform. That ultimately empowers all teams to control their software, but it also creates unique opportunities to measure the impact of the changes you’re making to your software.
When experiments are set up correctly, they provide valuable data to help inform your product decisions. But if you get something wrong, it could leave your team worse off than where you started.
That’s why properly selecting and allocating your audience is critical to achieving valid, informative results from experiments. For example, if you’re just consistently running experiments with an incorrect audience, it will inevitably lead to invalid data and in turn, guide your product team in the wrong direction.
Today, we’ve launched a major update to LaunchDarkly’s experimentation add-on that makes it simple to build and manage experiment audiences. This means any team can confidently set up valid experiments and focus on delivering the right value to customers.
Introducing the experiment allocation rule
Experiment traffic allocation is the process in which experimenters define what the audience for their experiment will be, how to assign the target audience to experiment variations, and which portion of that audience they wish to include in the analysis for an experiment’s reported results.
Allocation is also responsible for properly randomizing an experiment’s audience throughout the lifecycle of an experiment to ensure results are statistically unbiased and trustworthy.
In LaunchDarkly, audiences are defined using a feature flag’s highly customizable targeting rules.
In the past, experiments relied on experimenters knowing how to use a percent rollout to control users in each variation. This worked but required great care and knowledge to avoid common pitfalls.
Now, feature flags have an experiment allocation rule designed to provide experimenters a single place to define their target audience, select who is included for analysis, allocate their audience to the desired variations, and have everything properly randomized throughout the entirety of their experiment to ensure trustworthy results.
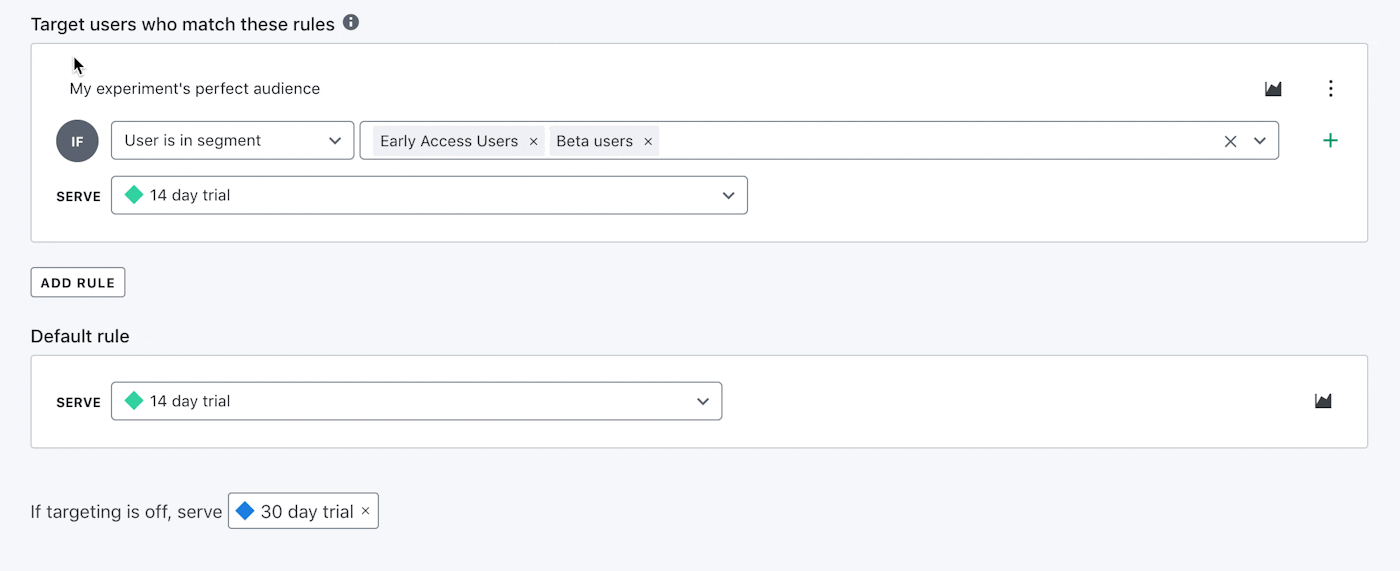
The new experiment allocation will also provide possible next steps for experimenters to breeze through setup and operation. If there are outstanding steps required for an experiment to be ready to run, we’ll pop a notice in the rule. When an experimenter ramps up the percent of traffic allocated to a variation, we will nudge them to reset their results to avoid time-based bias in their outcomes.
How does the experiment allocation rule help your team run better experiments?
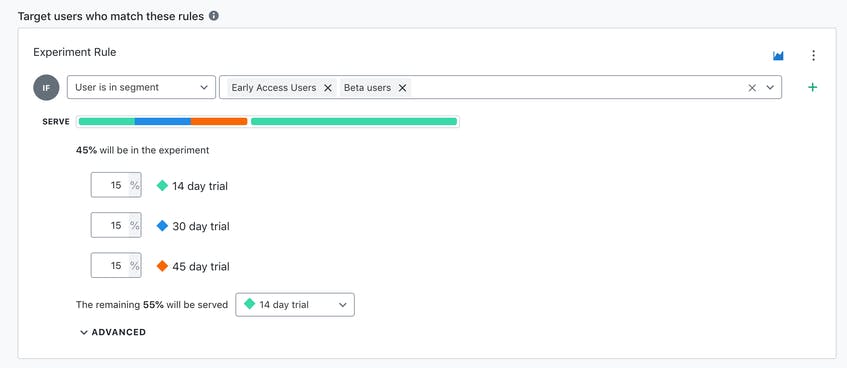
Prevents biased audience selection and allocation
With this update, LaunchDarkly now guides—and requires—that experiments will only allow a single experiment allocation rule to provide the audience for analysis.
Previously, it was too easy for experimenters to use multiple targeting rules in combinations that almost guaranteed bias in their results. Often these combinations are reasonable launch configurations for a business, but problematic to statistical analysis, and experimenters had to understand this to avoid bias.
Now, LaunchDarkly does the work for you to prevent imbalanced audiences, and ensures that your audiences are always properly selected and randomized to provide unbiased results.
Prevents carry-over bias when ramping down variations
Carry-over effects can invalidate experiment results when experimenters ramp down an underperforming variant and its traffic is not evenly re-distributed back to the remaining variants as the experiment continues onward.
When you use the experiment allocation rule type to operate an experiment, LaunchDarkly will now automatically rebalance and randomize users across your experiment’s variations so your results will always be balanced with no concern or effort.
Advanced controls enforce a consistent experience for experiment audiences
For some rare experiment designs, it’s important that users in the audience do not switch variations during the experiment. This does not include simple experiments like button color changes, but might include substantive changes, such as when Netflix switched from star ratings to thumbs up/down.
As part of the experiment allocation rule, LaunchDarkly now offers an advanced option to keep your experiment users assigned to the same variation throughout the lifetime of an experiment—which ensures a consistent user experience for the duration of the event.
With this capability, experimenters can configure their experiment’s variation assignment logic based on their experiment analysis needs and desired product behavior.
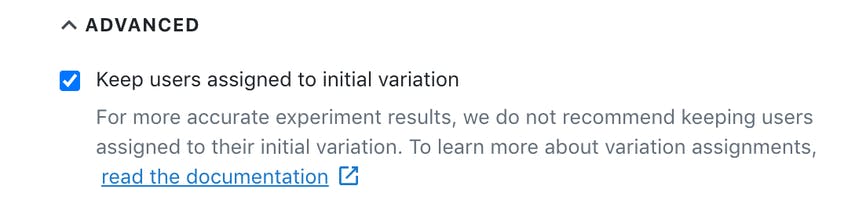
Easy control over experiment event volume costs
If your team has a large userbase, and experiment event volumes are a concern for costs, you may worry about how to run analysis on only a subset of your targeted audience to save money on total events.
Every experiment allocation setup provides full control over exactly what portion of the qualified audience will be included for analysis. It’s as simple as assigning a percentage of the total audience to each variation you want to include in the experiment. The remaining traffic will not be included in the experiment or results.
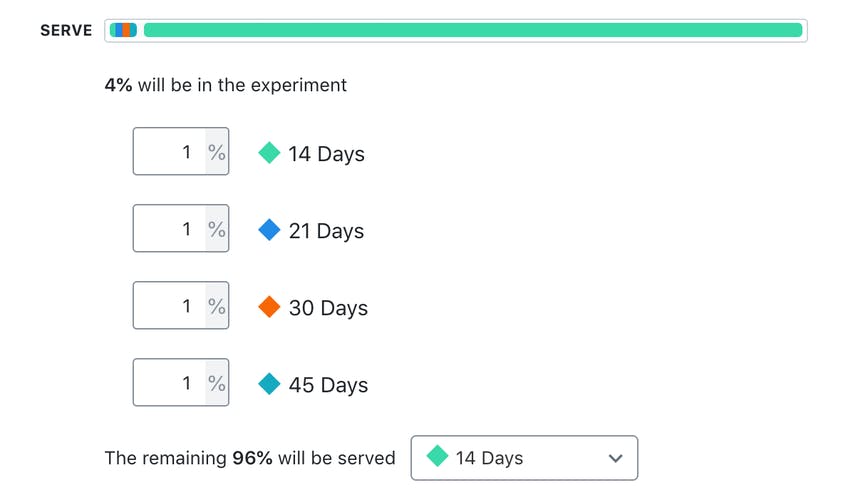
Helps team members complete experiment setup
An experiment requires both an audience from a flag and a metric to measure with. Previously, LaunchDarkly did little to ensure customers have set up both of these pieces before trying to run their experiment.
Many of our customers use our experimentation offering because they are not experts able to run complex experiments on their own. With the new experiment allocation rule type, LaunchDarkly will inform experimenters when they have an incomplete experiments setup and direct them to what needs to happen for their experiment to be ready to collect results.
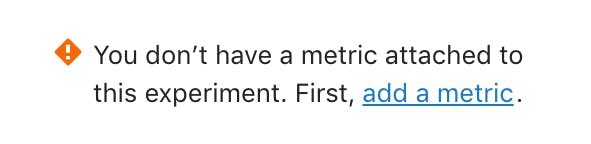
How can I get access to the new experiment allocation features?
Because this change requires some advanced changes to our bucketing logic, you must update your SDKs to the latest versions.
Once you’ve updated your SDKs, contact support@launchdarkly.com and we’ll enable it for you.
Will this affect any of my existing experiments?
No! When the new experiment allocation is enabled for your team, any running experiments will continue to function as normal. Only once an experiment is paused, will LaunchDarkly ask experimenters to transfer their audience designation over to the new rule type.
Can I run Multi-Armed Bandits in LaunchDarkly?
Yes! With our new traffic allocation feature we're able to support multi-armed bandit experiments. Read our best practices blog to learn more.
What’s next for LaunchDarkly experimentation?
At LaunchDarkly, our goal is to empower every team to deliver and control their software with confidence. Teams who can precisely measure the impact of their work will be able to make the right decision at the right time, and we will provide actionable analysis as part of any feature launch.
Up next, we’re making the entire experience of designing, operating, and acting on experiment outcomes even easier for any team member to make well informed decisions about a new release or KPI.
If you’d like to make smarter launch decisions with LaunchDarkly experimentation, contact our Sales team or your customer success rep. And as always, we’re always listening for your feedback at product@launchdarkly.com.