At LaunchDarkly, we believe experimentation is the key to innovationāexperimentation helpsĀ teams test, learn, and adapt faster than ever before. To make your experimentation projects more customizable, weāre thrilled to introduce the option to choose between Frequentist and Bayesian statistic models. This option gives your team the freedom to choose the statistical approach (Frequentist or Bayesian) that best suits their needs.
Whether you value the precision and familiarity of Frequentist methods or the dynamic insights of Bayesian analysis, you can now design and analyze experiments with confidence and clarity by choosing the model that works best for you making your experimentation with LaunchDarkly more accessible and flexible.
Why flexibility matters in experimentation
No two teams are alike when it comes to experimentation. Some are regular users of Frequentist statistics, leveraging fixed-horizon tests and p-values as standard practices. Others favor Bayesian approaches that use probabilities to guide iterative decisions. Some teams change their model based on the needs of a specific experiment.Ā
When tools force a one-size-fits-all model, it can create unnecessary friction. Imagine a team accustomed to Frequentist analysis encountering Bayesian probabilities, or a Bayesian-focused team suddenly working with p-values and statistical significance. A tool that forces one particular statistics model is sub-optimal for a team with differing needs and practices.
Introducing Frequentist and Bayesian statisticsĀ
Teams using LaunchDarkly can now select their preferred statistical framework when designing experiments. This flexibility lets teams:
- Use the methods they know best.
- Trust the results of experiments because they align with the organization's analytical framework.
- Eliminate friction to experimentation and begin leveraging data to drive decision-making.
Experiment builder enhancements
Now when you set out to create an experiment within LaunchDarkly, you will be prompted to:Ā
- Select your preferred analysis typeāFrequentist or Bayesianāwith a toggle.
- Provide key inputs for Frequentist experiments, including:
- Significance Level: Define the statistical rigor for your test.
- Test Direction: Choose between one-sided or two-sided tests.
- Set thresholds for Bayesian experiments, such as probability to beat baseline.
You can also use the LaunchDarkly Sample Size Calculator to determine the minimum necessary sample size or test duration to ensure that your experiment yields reliable results.
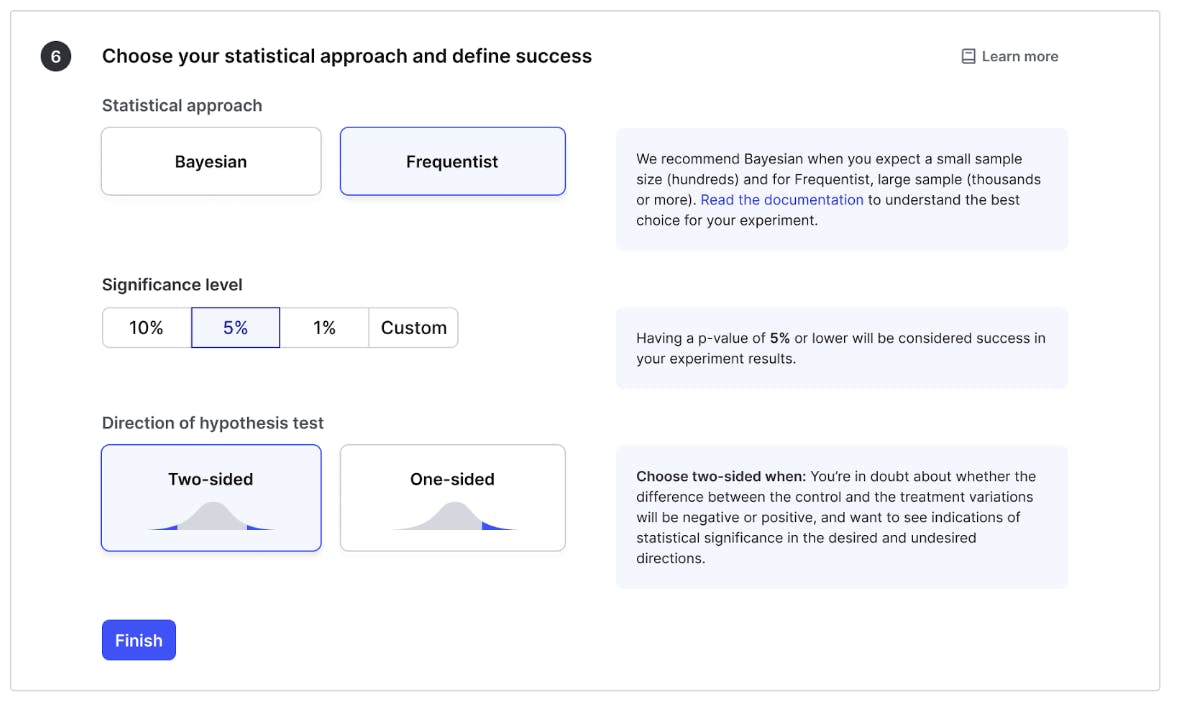
Choose between Bayesian and Frequentist when setting up your experiment in LaunchDarkly
Improved results presentation
After you start and run your experiment, youāll be able to view your results on the LaunchDarkly results page.Ā
- For Frequentist results, youāll now see:
- p-values for hypothesis testing
- Confidence intervals for deeper insights
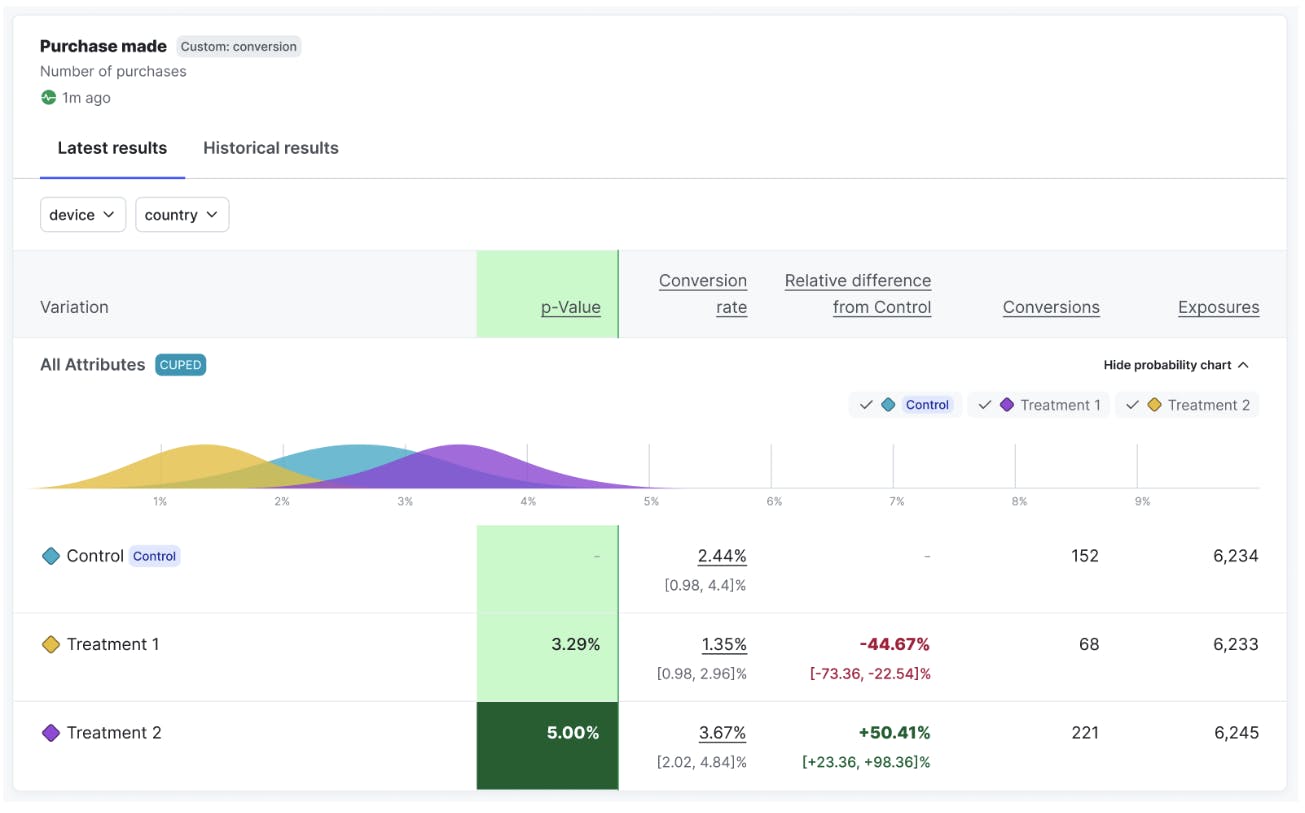
Frequentist experimentation results
- For Bayesian results, youāll now see:
- Probability to beat baseline
- Expected loss
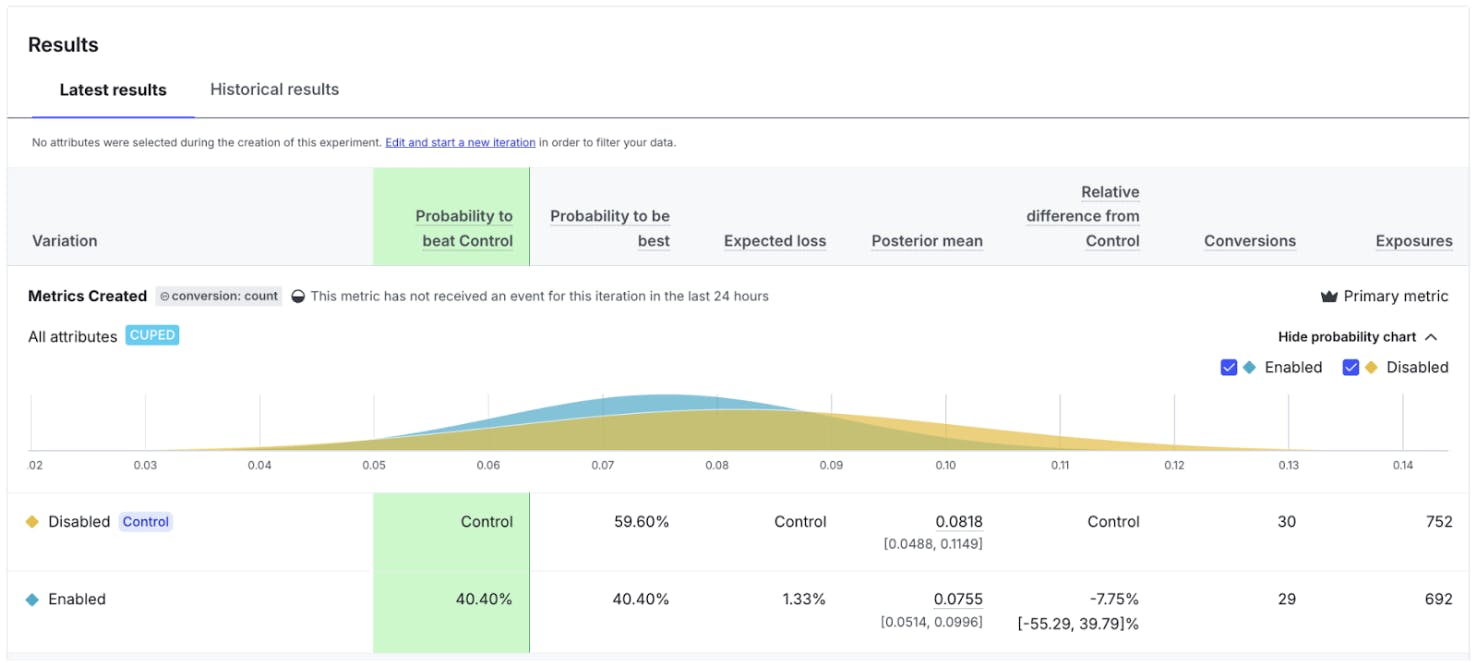
Bayesian experiment results
For other new additions to our experiments results page, check out our recent blog Introducing Enriched Experiment Results: Empowering Deeper Release Insights.
Get started with LaunchDarkly Experimentation today
Introducing the choice between Frequentist and Bayesian statistical models doesnāt just solve a technical challengeāit helps teams work more collaboratively. Using your preferred model allows product managers, experimenters, and data analysts to focus on interpreting results and driving action, instead of debating the benefits of statistical methodologies.
Already a LaunchDarkly Experimentation customer? Frequentist and Bayesian experiment types are now available to you! Learn more by checking out our product documentation or reaching out to your account manager.Ā
Interested in learning more about LaunchDarkly Experimentation? Sign up for a demo today!