In my role at LaunchDarkly, I'm fortunate enough to talk to many brilliant people across many different industries.
The stories I hear from the trenches occasionally strike a chord with me. The next thing I know, my fingers are flying so I can spread some of the gospel of experimentation.
I was talking with an unnamed leader at an unnamed organization about their frustration around sharing the results of an experiment with their business partners. They described their frustration this way:
You know, we did the right thing. We ran the experiment, and the results were pored over with an outrageous amount of scrutiny – likely because they didn’t like what the data was telling them. Do you know how many things were rolled out without any real measurement? And those are not held to this level of scrutiny! It’s like we’re being punished for providing the evidence.
For those of you in experimentation, this may sound familiar. Building a culture of experimentation often requires a rare skill set: the ability to gently push back on someone’s passion project and help them decouple the results of an experiment from the idea/hypothesis itself.
Put simply — no one likes it when you call their baby ugly. Even if it objectively is.
What is more compelling is that we know that as much as 90% of the time companies are inaccurate when they “trust their gut” on customer preferences.* That’s a lot of potentially ugly babies!
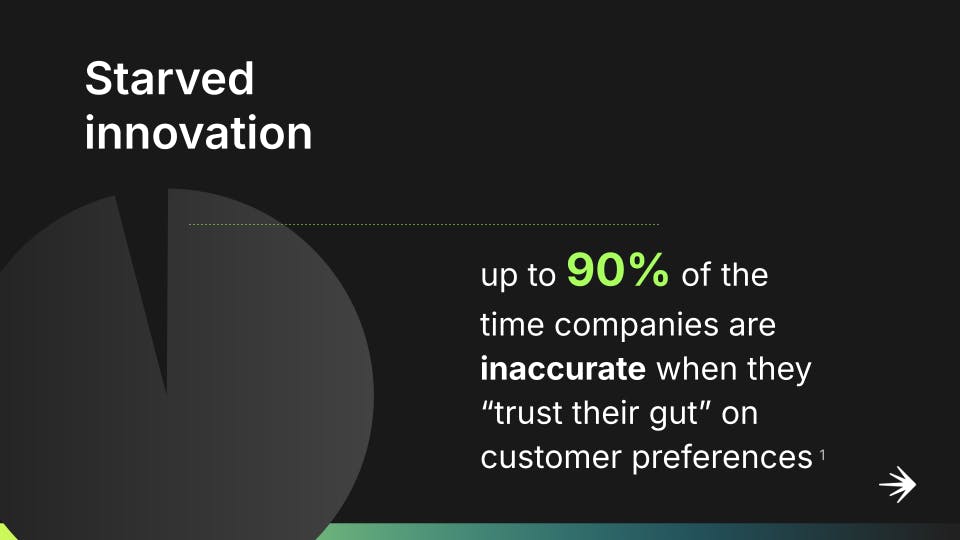
If up to 90% of decisions being made without an experiment are wrong, why are we not experimenting more? Or at the very least, why are we not at least spending more time questioning the results of every release? I mean, beside the fact that we’d grind all progress to a halt? Why do so many releases get a pass, while experiment results get torn into with meeting after meeting, new data pull after new data pull?
Don’t get me wrong; I love to scrutinize experiment results. One of my favorite things about running an experiment is discovering unexpected things, or developing new ideas as you really dig in and understand the data that is created. I want (and need) that level of scrutiny to be put towards every feature that is released!
I don’t want to paint an utterly dire picture here. More often than not, an experiment has a null result, or we don’t reach the level of confidence that we need to really trust that what we’re detecting is an actual signal and not just random noise. We can do the best experiment planning in the world, but the reason that we experiment is that real world data doesn’t always live up to our expectations. To steal a sports analogy – it’s why we play the game, right?
So the 90% statistic referenced above doesn’t mean that by running experiments, we’ll never make a bad decision again. But we can do so much more if we take just a little time to make sure that when we release something to market, we’re being thoughtful about how we’ll measure for success against our business metrics that matter.
Simply making sure you’re capturing the goals you want to drive when you release a feature to market is a great start. Actually running an experiment to measure the results of that feature is the gold standard! Releasing new features with careful planning in order to learn, adapt, and optimize your product, using real user data, is truly the only way to arm yourself with real information that you can use to make better decisions.
Experimentation doesn’t require massive changes to be worthwhile. In reality, experimentation is a way to validate and ensure that we’re making small, incremental improvements.
Let’s not get too ambitious here. Let’s imagine that by experimenting, we’re able to make just a 1% improvement on our business day over day, compared to a scenario where we’re “guessing” and getting the wrong answer, and a 1% decline in business day over day.
After time, that adds up. I’ll dig into this idea more soon, but for now: what are some things you might be able to do, as a diligent experimenter, to ensure that you don’t end up feeling like it would be easier to just give in and do a before/after measurement like everyone else?
Learn everything you can from your experiments
Learn what you can do on your next experiment that might help preempt this kind of deep scrutiny in the future. Run an experiment retrospective, some form of objective assessment of the execution and decision process for the experiment, to help ensure that the next experiment is smoother, faster, and cleaner; and is structured to make the next learning opportunity better.
Build a Hypo-Library
Build is likely too hefty a word for something that could be a simple shared notebook, list, Sharepoint site, or Slack channel. But when you come across something that needs further investigation, a new hypothesis to test—write it down! Make note of the circumstances that brought this idea to light, and take a decent swing at writing a hypothesis. It doesn’t need to be perfect, but the better you capture it in situ, before time and distance make you forget it, the more poised it’ll be to answer the question you really wanted answered!
Share your findings
I’m not suggesting walking directly out of a contentious meeting and crowing about how your results proved that so-and-so in <some other department> wrong.
But even if the findings of an experiment go against what you had predicted in your hypothesis, you have learned. And more than likely, you learned a number of things. What can others learn from your experiment, both from what you tested, but how you tested it? Help build that organizational experimentation muscle!
And take heart – even the smallest successes can lead to huge value over time! Stay tuned.
*A. Fabijan, P. Dmitriev, H. Olsson and J. Bosch, "The Benefits of Controlled Experimentation at Scale," in 2017 43rd Euromicro Conference on Software Engineering and Advanced Applications (SEAA), Vienna, Austria, 2017 pp. 18-26.